
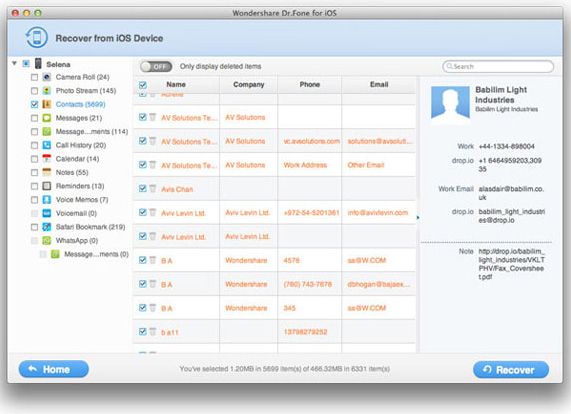
There were many other nice hacks and a good time was had by all. It was seriously impressive I hope he pushes it to matplotlib development. Theo went from not even knowing what an exoplanet is to doing this calculation in hours! Another impressive hack was Beaumont (Harvard), who hacked an OpenGL backend onto matplotlib, making the (notoriously slow but nice) plotting package outrageously fast for million-point plots. The most impressive was Schiminovich's 9-year-old son Theo's calculation of exoplanet transit lightcurves (including the secondary eclipse) in scratch. I wrote text, Foreman-Mackey wrote code, Cooper drew a graphical model, and Schwamb and Taub read papers. Foreman-Mackey and I (with help from Schwamb, Cooper, Taub) worked on a probabilistic analysis of exoplanet populations based on the Kepler objects of interest list. I had a great time at dotastronomy NYC hack day, organized by Muench (Harvard). Beautiful stuff, with good physics content and also very relevant to biological systems. He has also worked out the elasticity and normal modes of jammed soft particles (like cells). He has worked out quantitatively a thermodynamic analogy for non-thermal but randomly packed solids with some specific experimental systems. The second exam was a PhD defense for Ivane Jorjadze (NYU), who has various results on systems of jammed particles.

We vowed to discuss density estimation in January. He has lots of samples, but fitting the steady part (mean) of the spatially varying stochastic velocity distribution function is not trivial.
#VECTORIAL ASTROMETRY BY C A MURRAY PDF HOW TO#
One of the main problems he has is how to measure the drift velocity field of a particle, when the secular drift is a tiny, tiny fraction of the random velocity.
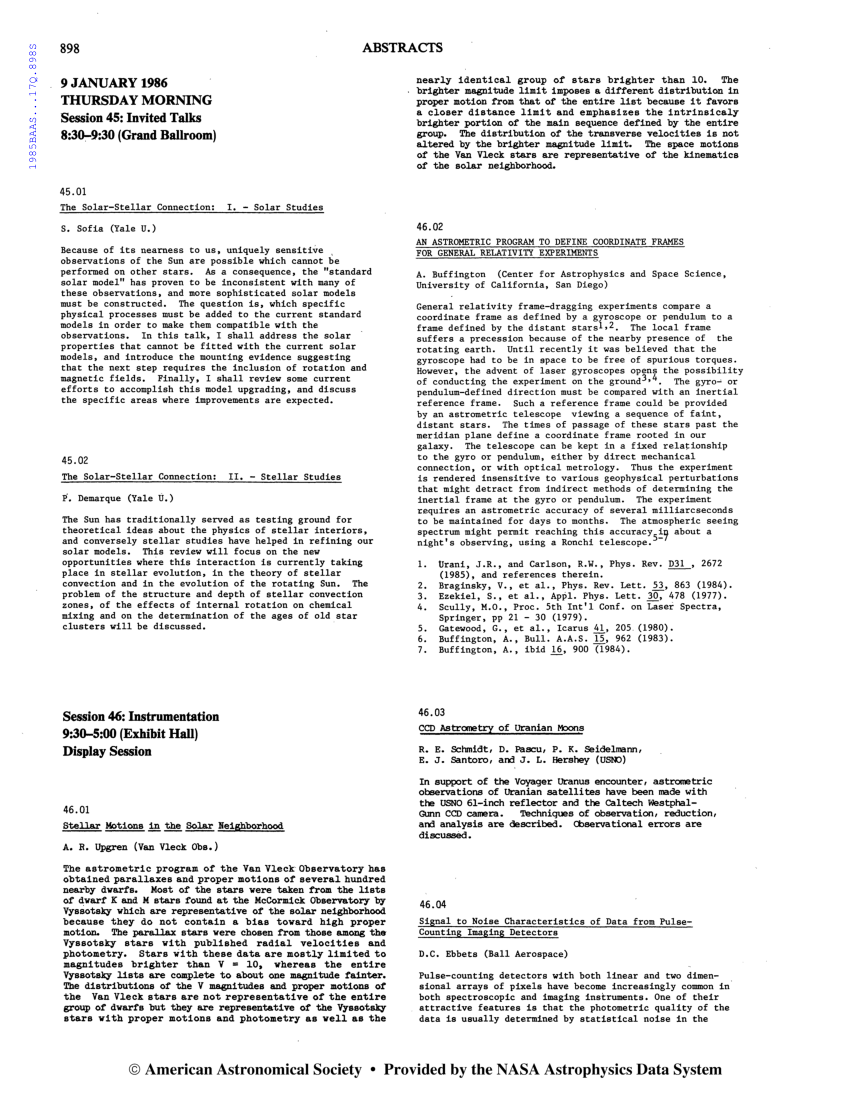
The first was a candidacy exam for Henrique Moyses (NYU), who is working on the stochastic vortex, a response of random-walking particles to non-conservative forces. I think we have resolved the differences that emerged during his talk here this semester. I spent a big chunk of the afternoon discussing gravitational radiation detection with Lam Hui (Columbia). We ended the talk with lunch, at which we asked Muandet to do some demos that elucidate the relationship between SVM and nearest neighbors (Fadely's current passion). Of course it has to differ, because it takes different inputs, but the question, correctly posed, is interesting. Jonathan Goodman asked how the SMM classifier differs from what you would get if you just ran SVM on a dense sampling of the input PDFs. I was pleased to see that the SMM contains, on the inside, something that looks very like chi-squareds or likelihood ratios. Muandet then went on to discuss his support measure machine extension of SVM in this extension the points are replaced by probability distribution functions (yes, the data are now PDFs). Indeed, the kernel obviates any creation of the higher dimensional space at all. For me the most valuable part of the discussion was an explanation of the kernel trick, which is absolutely brilliant: It projects the data up into a much higher-dimensional space and permits accurate classification without enormous computational load. Krik Muandet (MPI-IS) led a discussion today of support vector machines and extensions thereof. Like most visitors to Camp Hogg, he goes home with more unanswered questions than he arrived with, and new projects to boot! Today was also Muandet's last day here in NYC. I love that! He has the intuition that posterior predictive checks could revolutionize probabilistic inference with enormous data sets. We also discussed prior and posterior predictive checks, which Blei says he wants to do at scale and for science. Along the way, we learned that our confusion about how to treat model parameters that adjust model complexity arises in part because that genuinely is confusing. He softened when we explained that we can sample a big chunk of it already, but still expects that fully marginalizing out the exoplanets on the inside (we are trying to infer population properties at the top level of the hierarchy, using things like the Kepler data at the bottom level) will be impossible. Blei's initial reaction to our model is that it contains way too much data to be sampled and we will have to settle for optimization. In particular, we were interested in discussing what aspects of our planned exoplanet inferences will be tractable and what aspects will not, along with whether we can co-opt technology from other domains where complex graphical models have been sampled or marginalized. Camp Hogg met with David Blei (Princeton) again today to discuss some more the inference generalities that came up last week.
